1 Introduction
Pathogen contamination of food and water is a serious global challenge. About billion people do not have access to feces-free drinking water in the world111https://www.who.int/news-room/fact-sheets/detail/drinking-water. Such contaminated food and water can often lead to diarrhea. According to the World Health Organization (WHO), diarrhoeal diseases account for the loss of around half a million human lives annually. Similarly, nearly million people fall ill due to the consumption of contaminated food, resulting in more than two hundred thousand deaths every year222https://www.who.int/news-room/fact-sheets/detail/food-safety. Giardia and Cryptosporidium are two of the major causes of protozoan-induced diarrheal diseases and are the most frequently identified protozoan parasites causing outbreaks (Baldursson and Karanis, 2011)††footnotemark: . In low- and middle-income countries, children under three years of age experience three episodes of diarrhea on average every year333https://www.who.int/news-room/fact-sheets/detail/diarrhoeal-disease. Giardia and Cryptosporidium cause intestinal illness called giardiasis and cryptosporidiosis, respectively. Infections due to Cryptosporidium and Giardia are more common in low- and middle-income countries because of unhygienic lifestyles, and poor sanitation (Fricker et al., 2002; Gupta et al., 2020; Tandukar et al., 2013; Sherchand et al., 2004). Giardia is more prominent in size with an elliptical shape having a major axis 8-12 µm and minor axis 7-15 µm. In contrast, Cryptosporidium is spherically shaped, having a diameter of 3-6 µm (Dixon et al., 2011). Accurate detection of these microorganisms could enable early diagnosis, saving millions of lives. Moreover, regularly screening these pathogens in real food and water samples could help prevent infections and disease outbreaks.
Polymerase chain reaction (PCR), immunological assays, cell culture methods, fluorescence in situ hybridization, and microscopic analysis (Van den Bossche et al., 2015; Adeyemo et al., 2018) are the main methods for the detection of (oo)cysts of Giardia and Cryptosporidium. Even though these methods are reliable and accurate, they are laborious, time-consuming, costly, and require significant expertise, resulting in a lack of tests needed in many resource-limited regions. For instance, cell culture requires more than hours, and immunological assays and PCR reactions require costly reagents and equipment (Guerrant et al., 1985, 2001). In addition, some viable bacterial pathogens are difficult to grow or are even non-culturable (Oliver, 2005). Fluorescence tagging of cells requires expertise, and the cost of reagents is high. Microscopic methods are the most widely used diagnostic methods for detecting parasites, especially in low-resource countries. The microscopic method examines a glass slide containing a sample under a microscope. However, the traditional microscopes are still costly, less portable, and require expertise to handle and accurately identify microorganisms on the slides (Chavan and Sutkar, 2014). Recently, smartphone-based microscopic methods have been developed to potentially replace or supplement more expensive and less portable microscopic methods, including the brightfield and fluorescence microscopy (Koydemir et al., 2015; Kobori et al., 2016; Kim et al., 2015; Saeed and Jabbar, 2018; Shrestha et al., 2020; Feng et al., 2016). These microscopes allow magnification of microorganisms enabling the user to observe them on the phone screen immediately and capture the images and videos using the smartphone. However, smartphone microscopy also requires a well-trained person to identify target organisms accurately, analyze the result, and report it. In the least developed countries, the lack of skilled technicians limits the use of such a new microscopic system for rapid field testing and clinical applications. Therefore, robust and automated detection of microorganisms using smartphone microscopic images could enable more widespread use of smartphone microscopes in large-scale screening of parasites.
In recent years, several deep learning-based algorithms have been developed for various biological and clinical applications such as automatic detection, segmentation, and classification of human cells and fungi species (Xue and Ray, 2017; Zieliński et al., 2020), bacteria (Wang et al., 2020), malaria detection (Vijayalakshmi et al., 2020), image segmentation of two-dimensional materials in microscopic images (Masubuchi et al., 2020). In addition, detecting a pollen grain from microscopy images using a deep neural network has been reported (Gallardo-Caballero et al., 2019). de Haan et al. (2020) automated the screening of sickle cells using deep learning on a smartphone-based microscope. Similarly, Xu et al. (2020) proposed ParasNet - a deep-learning-based network - to detect Giardia and Cryptosporidium in brightfield microscopic images. Luo et al. (2021) created MCellNet to classify Giardia, Cryptosporidium, microbeads, and natural pollutants from the images captured from imaging flow cytometry. Machine learning techniques have also been developed to classify Giardia from other parasites using features such as area, equivalent diameter, and intensity in fluorescent smartphone-based microscopic images (Koydemir et al., 2015). Several other studies have proposed deep learning-based algorithms to automate microorganism detection in smartphone microscopes (de Haan et al., 2020; Fuhad et al., 2020; Yang et al., 2019). However, it is not clear how well state-of-the-art deep learning models perform in automated detection of the (oo)cysts of Giardia and Cryptosporidium from smartphone microscopic images in comparison to the traditional microscope and non-experts. We also introduce labeled smartphone and brightfield microscopy datasets for detection of the (oo) cysts of Giardia and Cryptosporidium, making it publicly available for the scientific community.
Here, we explore the potential of deep learning algorithms to detect cysts of two different kinds of enteric parasites without human experts’ involvement in images taken using a custom-built smartphone microscopy system. We created a custom dataset by capturing images of sample slides from a smartphone microscope and a traditional brightfield microscope. The sample slides were obtained for (oo)cysts on both - reference and vegetable sample extracts. We trained three popular object detection models - Faster RCNN (Ren et al., 2016), RetinaNet (Lin et al., 2017), and YOLOv8s (Jocher et al., 2023) using our dataset and evaluated the performance of the models. Finally, we report the performance of these models compared with expert humans and non-expert humans and inter-operator variability. The performance of the models with smartphone microscopic images was also compared with brightfield microscopic images. The dataset and the source code are made publicly available.
2 Methods
2.1 Dataset
2.1.1 Training-Validation Set
We used two microscopes to capture images: i) a traditional brightfield microscope and ii) a sapphire ball lens-based smartphone microscope developed by Shrestha et al. (2020). The brightfield microscope images were captured with a rectangular field of view (FOV) of 190 µm X µm and a magnification of 400X. In contrast, the smartphone microscope images were captured with a circular FOV of diameter µm and magnification of 200X.
We captured images by making microscope slides from two different types of samples: i) standard or reference (oo)cyst suspension samples (Waterborne Inc, PC101 G/C positive control), and ii) actual vegetable samples obtained from local markets in Nepal. Figure 1 shows a few examples of reference and actual vegetable samples along with their bounding box annotations. Likewise, Table 1 summarizes the number and types of images captured in the dataset.
Reference Samples: We prepared slides each from µL standard (oo)cyst suspension (or standard samples) mixed with Lugol’s iodine in equal proportion. From these slides, an expert with more than two years of experience in imaging and annotating Giardia and Cryptosporidium from microscopy images captured many images from both microscopes. To maintain consistency, we selected best images, each based on the clarity of parasites. Since there was no prior knowledge of parasite counts in individual slides, identification and counting of (oo)cysts by the expert was considered as a benchmark.
Vegetable Samples: We prepared slides from vegetable samples collected from local markets in Nepal. The expert captured and selected images using the same protocol as the one used for the reference samples. From these slides, 1005 images for each type of microscope were included for further steps. The images were used for training the object detection models.
2.1.2 Independent Test Set
To assess the generalization of deep learning models on smartphone microscope images, an independent test set was prepared as follows: One hundred ninety-three images were captured on a particular day using the same smartphone microscope. The expert captured images at random locations of the microscope slides, regardless of the presence of parasites. The expert annotated these images with bounding boxes and ellipses for all Giardia and Cryptosporidium using VGG annotator (Dutta and Zisserman, 2019).
2.1.3 Non-Expert Annotated Set
The expert trained three non-expert humans on smartphone vegetable sample images for three hours. The non-experts were instructed to use the shape and size for identifying the (oo)cysts of Giardia and Cryptosporidium. The three non-experts annotated the two types of cysts in images of the independent test set. Non-experts used MS-paint for annotations by encircling the cysts of the two parasites with ellipses of two different colors, and the time non-experts took to annotate the cysts in each of the images was recorded using a stopwatch.

Dataset | No. of images | Microscopes | Giardia’s annotation | Cryptosporidium’s annotation |
Reference sample | Smartphone | |||
Brightfield | ||||
Vegetable sample | Smartphone | |||
Brightfield | ||||
Test (vegetable sample) | Smartphone |
2.2 Deep Learning-based Object Detection Models
Three state-of-the-art object detection models were selected for this study: Faster RCNN (Ren et al., 2016), RetinaNet (Lin et al., 2017), and YOLOv8s (Jocher et al., 2023). Faster RCNN is one of the most popular networks from the Region-Based Convolutional Neural Networks (RCNN) family, which is an improved version based on two previous methods, RCNN (Girshick et al., 2014) and Fast RCNN (Girshick, 2015). RetinaNet is a popular single-stage detector that uses a Focal Loss to address the foreground-background class imbalance problem that gets more severe when detecting smaller objects in the images. YOLOv8s is a popular single-stage detector with relatively low computational costs enabling it to be run on smartphones. To assess the quality of the real-time detection model that can be run on smartphones, we chose YOLOv8s (smallest model with million parameters) from the various available models for YOLOv8s, as it is lightweight and has the lowest prediction time (Jocher et al., 2023).
2.3 Experimental Setup
Figure 2 illustrates the overall pipeline of the training and evaluation of the three object detectors using expert and non-expert annotated images captured from brightfield and smartphone images. During training, the images were first pre-processed (see subsection A.1) and then fed as an input to one of the three object detection models for classifying the object type (Giardia or Cryptosporidium) and localizing the objects with a bounding box.

2.3.1 Evaluation approach
Since the target application is to be able to assess the contamination in vegetable samples by identifying and counting the number of (oo)cysts of the two parasites in the microscopic images, we evaluate the three models using classification performance metrics: precision, recall, and F1 score. The model-predicted cysts can belong to one of the three categories: true positive (TP) when the model’s object prediction (of either Giardia or Cryptosporidium cysts) correctly matches with the Ground Truth (GT) annotation, false positive (FP) when the model’s object prediction is different than the GT, and finally false negative (FN) when the model does not detect cysts annotated by the experts in the images. Precision, Recall, and F1 score are calculated based on these three values to evaluate the models’ performances.
(1) |
(2) |
(3) |
In addition to assessing the object detection model’s performance against the expert GT annotations, we also evaluate how the model compares with non-expert humans. This helps assess the utility of deploying the automated models in places where the experts are not available.
2.3.2 Comparing the three models and non-expert humans
We evaluated the object detection models - Faster RCNN, RetinaNet, and YOLOv8s - in the following settings:
- •
5-fold cross-validation of the brightfield and smartphone microscope images using the training-validation dataset with expert annotations: 830X2 images for the two microscopes with reference and 1005X2 images with vegetable samples.
- •
Comparison of the detection models against non-expert humans in identifying the (oo)cysts of the two parasites in separate independent smartphone microscope test images (n=193).
- •
Comparison of time taken by non-experts vs. detection model to identify the (oo)cysts in the smartphone microscope test images.
To test whether the differences in the performance of the non-expert humans and the detection models in the independent test set are statistically significant, we use paired Wilcoxon signed-rank test (Woolson, 2007). The details are provided in subsection A.2.
3 Results
3.1 5-fold Cross-validation in training-validation set
Table 2 presents the performance of the three object detection models for the brightfield microscope images of reference and vegetable samples. The results are reported for confidence scores() and iou-thresholds() optimized for each model separately. The details about the choice of these thresholds are provided in subsection A.3. We see that the models perform better for reference samples than vegetable samples. It is expected because the reference sample does not have debris, and hence there are very few objects confounding with the cysts in the clean brightfield images (example images in Figure 1). Similarly, we observe that all the object detectors detect Giardia cysts better than Cryptosporidium ones, except for Faster RCNN which has negligibly better performance in detecting Cryptosporidium.
Dataset | Models | Thresholds | Precision | Recall | F1-score |
---|---|---|---|---|---|
Giardia | |||||
Reference Sample | Faster RCNN | ||||
RetinaNet | |||||
YOLOv8s | |||||
Vegetable Sample | Faster RCNN | 0.026 | |||
RetinaNet | |||||
YOLOv8s | |||||
Cryptosporidium | |||||
Reference Sample | Faster RCNN | ||||
RetinaNet | |||||
YOLOv8s | |||||
Vegetable Sample | Faster RCNN | 0.045 | 0.8390.024 | ||
RetinaNet | |||||
YOLOv8s |
Table 3 shows 5-fold cross-validation results for Smartphone microscope training-validation set images. As expected, the object detectors’ performance is lower than brightfield microscope images, because smartphone microscope images have more textured noise and lower magnification than traditional brightfield microscopes. We see that most of the trends observed in brightfield microscope images can also be seen for smartphone microscope: Giardia cysts are better detected than Cryptosporidium cysts, YOLOv8s and Faster RCNN provide better results than RetinaNet, except for vegetable samples, and the performance of the detectors is better for reference samples compared to the vegetable sample in general. However, one notable exception is that the F1-score for the vegetable sample is better than the reference sample for Cryptosporidium when using YOLOv8s. In this case, while the recall reduced slightly in the vegetable sample, the precision increased substantially, increasing the overall F1 score. RetinaNet provides better results in detecting Giardia and Cryptosporidium in vegetable samples, but Faster RCNN is better for reference samples.
Dataset | Models | Thresholds | Precision | Recall | F1-score |
---|---|---|---|---|---|
Giardia | |||||
Reference Sample | Faster RCNN | ||||
RetinaNet | |||||
YOLOv8s | |||||
Vegetable Sample | Faster RCNN | ||||
RetinaNet | |||||
YOLOv8s | |||||
Cryptosporidium | |||||
Reference Sample | Faster RCNN | ||||
RetinaNet | |||||
YOLOv8s | |||||
Vegetable Sample | Faster RCNN | ||||
RetinaNet | |||||
YOLOv8s |
3.2 Independent Test Set with Smartphone Microscope Images
Table 4 presents the performance of the three models and non-expert humans on the independent test set images. This set consists of smartphone microscopic images of vegetable samples where the expert’s annotation is considered ground truth. The performance of all the models on the test set images is lower than that of cross-validation, which is typical for machine learning models, known as the generalization problem. YOLOv8s, which performed well in cross-validation, has the worst overall score in the test set, suggesting that the model was less robust to new data. In contrast, the RetinaNet model is more robust to the new data. Moreover, RetinaNet seems to perform better for Cryptosporidium, which could be due to the Focal Loss targeted for smaller objects, as Cryptosporidium cysts have a smaller size than Giardia cysts. Nevertheless, the test set results reinforce the observation that detecting Cryptosporidium is more difficult compared to Giardia cysts.
Detector | Giardia | Cryptosporidium | ||||
---|---|---|---|---|---|---|
Precision | Recall | F1-score | Precision | Recall | F1-score | |
Faster RCNN | ||||||
RetinaNet | ||||||
YOLOv8s | ||||||
Non-expert1 | ||||||
Non-expert2 | ||||||
Non-expert3 |
Wilcoxon signed-rank test showed that all the models and non-expert humans mostly had significantly different results, except for Non-expert , who had non-significant statistical results with Faster RCNN and RetinaNet, for both Giardia and Cryptosporidium. (Details provided in subsection A.2.)
Since the ability to detect the cysts in real-time using only the smartphone can be valuable for rapid field testing scenarios, we computed the time required to detect the cysts for the different models and the human experts and non-experts. YOLOv8s was the fastest, predicting the objects in an average of seconds per image, whereas Faster RCNN and RetinaNet needed seconds and seconds, respectively. Among the human annotators, the expert identified cysts in seconds on average per image, but the non-experts took as long as seconds. FasterRCNN, RetinaNet, and YOLOv8s comprise , , and million parameters, respectively.
4 Discussion and Conclusion
In this study, we explored the possibility of using automatic parasite detection in brightfield and smartphone microscopes for the (oo)cysts of Giardia and Cryptosporidium in scenarios where experts are not available. Two different datasets were prepared by separately capturing the images of reference and actual vegetable samples using the smartphone and the brightfield microscopes. Three object detection models were explored, and their performance was compared against human non-experts while taking an expert annotation as ground truth. Precision, recall, and F1 scores were used as they are useful evaluation metrics when a target application requires counting objects (Xue and Ray, 2017; Brhane Hagos et al., 2019).
The results show that for the same range of training samples models perform better on reference samples than vegetable samples, brightfield microscopes than smartphone microscopes, Giardia than Cryptosporidium cysts. Vegetable samples have debris similar to Giardia and Cryptosporidium (Figure 1) making the task more difficult. Smartphone images are more textured and noisy. Additionally, due to the curvature effect of the ball lens in the smartphone microscope, the objects get stretched toward the peripheral regions and appear bigger than those at the center of the image. In such cases, the models could falsely predict Giardia as Cryptosporidium and vice versa, as shown in Figure 3. A larger annotated dataset and providing global context by using transformer architectures instead of CNNs could in the future enable models to correctly identify such stretched objects. Some smartphone images seem to be blurry, which causes false negatives as shown in Figure 3a. Figure 3c illustrates a scenario of multiple predictions where both classes were predicted for a single object. However, it was only observed when using RetinaNet. In other models, the problem was eliminated by increasing the confidence threshold level for the prediction. Giardia was better predicted by all three models used in the study, which might be due to its larger size and ellipse shape. Future works can also focus on developing robust models in detecting tiny Cryptosporidium cysts.
This work shows the feasibility and promise of integrating deep learning-based automated models into brightfield and smartphone microscopes, especially in resource-constrained areas where experts are not readily available. Although the models performed better than non-experts in Cryptosporidium cysts, the F1-scores for the models are still relatively low in test-sets. Future work requires collecting a much larger dataset, which will improve the scores. Similarly, assessing the variability among the experts by annotating a certain subset and comparing this variability against AI models would also be interesting. A larger dataset can be used for self-supervised pertaining on unlabeled samples, followed by a supervised fine-tuning on annotated dataset to get better object detection performance. Moreover, in this study, we have not combined reference and vegetable sample images to train the models. The mixed dataset could be used in future works hoping for better performance and domain adaptation.

In our experiments, YOLOv8s has decent performance in the real vegetable samples. Considering its lightweight architecture (22 MB), and good performance in detecting the cysts, YOLOv8s shows potential to be deployed on mobile devices without the need for a server (Kuznetsova et al., 2021; Chen et al., 2021).
This work provides the first step and shows the feasibility of a low-cost smartphone-based automated detection of (oo)cysts or other microorganisms in vegetables, water, stool, or other food products without the need for an expert. More than specific deep learning models to choose, future work should focus on larger dataset or semi-supervised approaches, and designing experiments in prospective settings to compare against non-experts and experts for diagnostic end-points.
5 Data and Code
The data and code for the experiments are available at https://github.com/naamiinepal/smartphone_microscopy.
Acknowledgments
We thank Rabin Adhikari for helping us with formatting. The images used in this research were taken with support from NAS and USAID (to BG and BBN) through Partnerships for Enhanced Engagement in Research (PEER) (AIDOAA-A-11-00012). The opinions, findings, conclusions, or recommendations expressed in this article are those of the authors alone and do not necessarily reflect the views of USAID or NAS.
Ethical Standards
The work follows appropriate ethical standards in conducting research and writing the manuscript, following all applicable laws and regulations regarding the treatment of animals or human subjects.
Conflicts of Interest
The authors declare no conflicts of interest.
References
- Adeyemo et al. (2018) Folasade Esther Adeyemo, Gulshan Singh, Poovendhree Reddy, and Thor Axel Stenström. Methods for the detection of cryptosporidium and giardia: from microscopy to nucleic acid based tools in clinical and environmental regimes. Acta tropica, 184:15–28, 2018.
- Baldursson and Karanis (2011) Selma Baldursson and Panagiotis Karanis. Waterborne transmission of protozoan parasites: review of worldwide outbreaks–an update 2004–2010. Water research, 45(20):6603–6614, 2011.
- Brhane Hagos et al. (2019) Yeman Brhane Hagos, Priya Lakshmi Narayanan, Ayse U Akarca, Teresa Marafioti, and Yinyin Yuan. Concorde-net: Cell count regularized convolutional neural network for cell detection in multiplex immunohistochemistry images. arXiv e-prints, pages arXiv–1908, 2019.
- Chavan and Sutkar (2014) Sneha Narayan Chavan and Ashok Manikchand Sutkar. Malaria disease identification and analysis using image processing. Int. J. Latest Trends Eng. Technol, 3(3):218–223, 2014.
- Chen et al. (2019) Kai Chen, Jiaqi Wang, Jiangmiao Pang, Yuhang Cao, Yu Xiong, Xiaoxiao Li, Shuyang Sun, Wansen Feng, Ziwei Liu, Jiarui Xu, et al. Mmdetection: Open mmlab detection toolbox and benchmark. arXiv preprint arXiv:1906.07155, 2019.
- Chen et al. (2021) Yuwen Chen, Chao Zhang, Tengfei Qiao, Jianlin Xiong, and Bin Liu. Ship detection in optical sensing images based on yolov5. In Twelfth International Conference on Graphics and Image Processing (ICGIP 2020), volume 11720, page 117200E. International Society for Optics and Photonics, 2021.
- de Haan et al. (2020) Kevin de Haan, Hatice Ceylan Koydemir, Yair Rivenson, Derek Tseng, Elizabeth Van Dyne, Lissette Bakic, Doruk Karinca, Kyle Liang, Megha Ilango, Esin Gumustekin, et al. Automated screening of sickle cells using a smartphone-based microscope and deep learning. NPJ Digital Medicine, 3(1):1–9, 2020.
- Dixon et al. (2011) BR Dixon, Ronald Fayer, Mónica Santín, DE Hill, and JP Dubey. Protozoan parasites: Cryptosporidium, giardia, cyclospora, and toxoplasma. Rapid detection, characterization, and enumeration of foodborne pathogens, pages 349–370, 2011.
- Dutta and Zisserman (2019) Abhishek Dutta and Andrew Zisserman. The VIA annotation software for images, audio and video. In Proceedings of the 27th ACM International Conference on Multimedia, MM ’19, New York, NY, USA, 2019. ACM. ISBN 978-1-4503-6889-6/19/10. doi: 10.1145/3343031.3350535. URL https://doi.org/10.1145/3343031.3350535.
- Feng et al. (2016) Steve Feng, Derek Tseng, Dino Di Carlo, Omai B Garner, and Aydogan Ozcan. High-throughput and automated diagnosis of antimicrobial resistance using a cost-effective cellphone-based micro-plate reader. Scientific reports, 6(1):1–9, 2016.
- Fricker et al. (2002) CR Fricker, GD Medema, and HV Smith. Protozoan parasites (cryptosporidium, giardia, cyclospora). Guidelines for drinking-water quality, 2:70–118, 2002.
- Fuhad et al. (2020) KM Fuhad, Jannat Ferdousey Tuba, Md Sarker, Rabiul Ali, Sifat Momen, Nabeel Mohammed, and Tanzilur Rahman. Deep learning based automatic malaria parasite detection from blood smear and its smartphone based application. Diagnostics, 10(5):329, 2020.
- Gallardo-Caballero et al. (2019) Ramón Gallardo-Caballero, Carlos J García-Orellana, Antonio García-Manso, Horacio M González-Velasco, Rafael Tormo-Molina, and Miguel Macías-Macías. Precise pollen grain detection in bright field microscopy using deep learning techniques. Sensors, 19(16):3583, 2019.
- Girshick (2015) Ross Girshick. Fast r-cnn. In Proceedings of the IEEE international conference on computer vision, pages 1440–1448, 2015.
- Girshick et al. (2014) Ross Girshick, Jeff Donahue, Trevor Darrell, and Jitendra Malik. Rich feature hierarchies for accurate object detection and semantic segmentation. In Proceedings of the IEEE conference on computer vision and pattern recognition, pages 580–587, 2014.
- Guerrant et al. (1985) Richard L Guerrant, David S Shields, Stephen M Thorson, John B Schorling, and Dieter HM Groschel. Evaluation and diagnosis of acute infectious diarrhea. The American journal of medicine, 78(6):91–98, 1985.
- Guerrant et al. (2001) Richard L Guerrant, Thomas Van Gilder, Ted S Steiner, Nathan M Thielman, Laurence Slutsker, Robert V Tauxe, Thomas Hennessy, Patricia M Griffin, Herbert DuPont, R Bradley Sack, et al. Practice guidelines for the management of infectious diarrhea. Clinical infectious diseases, 32(3):331–351, 2001.
- Gupta et al. (2020) Ranjit Gupta, Binod Rayamajhee, Samendra P Sherchan, Ganesh Rai, Reena Kiran Mukhiya, Binod Khanal, and Shiba Kumar Rai. Prevalence of intestinal parasitosis and associated risk factors among school children of saptari district, nepal: a cross-sectional study. Tropical medicine and health, 48(1):1–9, 2020.
- Jocher et al. (2023) Glenn Jocher, Ayush Chaurasia, and Jing Qiu. YOLO by Ultralytics, 1 2023. URL https://github.com/ultralytics/ultralytics.
- Kim et al. (2015) Jung-Hyun Kim, Hong-Gu Joo, Tae-Hoon Kim, and Young-Gu Ju. A smartphone-based fluorescence microscope utilizing an external phone camera lens module. BioChip Journal, 9(4):285–292, 2015.
- Kobori et al. (2016) Yoshitomo Kobori, Peter Pfanner, Gail S Prins, and Craig Niederberger. Novel device for male infertility screening with single-ball lens microscope and smartphone. Fertility and sterility, 106(3):574–578, 2016.
- Koydemir et al. (2015) Hatice Ceylan Koydemir, Zoltan Gorocs, Derek Tseng, Bingen Cortazar, Steve Feng, Raymond Yan Lok Chan, Jordi Burbano, Euan McLeod, and Aydogan Ozcan. Rapid imaging, detection and quantification of giardia lamblia cysts using mobile-phone based fluorescent microscopy and machine learning. Lab on a Chip, 15(5):1284–1293, 2015.
- Kuznetsova et al. (2021) Anna Kuznetsova, Tatiana Maleva, and Vladimir Soloviev. Yolov5 versus yolov3 for apple detection. Cyber-Physical Systems: Modelling and Intelligent Control, pages 349–358, 2021.
- Lin et al. (2017) Tsung-Yi Lin, Priya Goyal, Ross Girshick, Kaiming He, and Piotr Dollár. Focal loss for dense object detection. In Proceedings of the IEEE international conference on computer vision, pages 2980–2988, 2017.
- Luo et al. (2021) Shaobo Luo, Kim Truc Nguyen, Binh TT Nguyen, Shilun Feng, Yuzhi Shi, Ahmed Elsayed, Yi Zhang, Xiaohong Zhou, Bihan Wen, Giovanni Chierchia, et al. Deep learning-enabled imaging flow cytometry for high-speed cryptosporidium and giardia detection. Cytometry Part A, 2021.
- Masubuchi et al. (2020) Satoru Masubuchi, Eisuke Watanabe, Yuta Seo, Shota Okazaki, Takao Sasagawa, Kenji Watanabe, Takashi Taniguchi, and Tomoki Machida. Deep-learning-based image segmentation integrated with optical microscopy for automatically searching for two-dimensional materials. npj 2D Materials and Applications, 4(1):1–9, 2020.
- Nguyen et al. (2020) Nhat-Duy Nguyen, Tien Do, Thanh Duc Ngo, and Duy-Dinh Le. An evaluation of deep learning methods for small object detection. Journal of Electrical and Computer Engineering, 2020, 2020.
- Oliver (2005) James D Oliver. The viable but nonculturable state in bacteria. Journal of microbiology, 43(spc1):93–100, 2005.
- Ren et al. (2016) Shaoqing Ren, Kaiming He, Ross Girshick, and Jian Sun. Faster r-cnn: Towards real-time object detection with region proposal networks. IEEE transactions on pattern analysis and machine intelligence, 39(6):1137–1149, 2016.
- Saeed and Jabbar (2018) Muhammad A Saeed and Abdul Jabbar. “smart diagnosis” of parasitic diseases by use of smartphones. Journal of clinical microbiology, 56(1), 2018.
- Sherchand et al. (2004) JB Sherchand, PN Misra, DN Dhakal, et al. Cryptosporidium parvum: An observational study in kanti children hospital, kathmandu, nepal. Journal of Nepal Health Research Council, 2004.
- Shrestha et al. (2020) Retina Shrestha, Rojina Duwal, Sajeev Wagle, Samiksha Pokhrel, Basant Giri, and Bhanu Bhakta Neupane. A smartphone microscopic method for simultaneous detection of (oo) cysts of cryptosporidium and giardia. PLoS Neglected Tropical Diseases, 14(9), 2020.
- Tandukar et al. (2013) Sarmila Tandukar, Shamshul Ansari, Nabaraj Adhikari, Anisha Shrestha, Jyotshana Gautam, Binita Sharma, Deepak Rajbhandari, Shikshya Gautam, Hari Prasad Nepal, and Jeevan B Sherchand. Intestinal parasitosis in school children of lalitpur district of nepal. BMC research notes, 6(1):449, 2013.
- Van den Bossche et al. (2015) Dorien Van den Bossche, Lieselotte Cnops, Jacob Verschueren, and Marjan Van Esbroeck. Comparison of four rapid diagnostic tests, elisa, microscopy and pcr for the detection of giardia lamblia, cryptosporidium spp. and entamoeba histolytica in feces. Journal of microbiological methods, 110:78–84, 2015.
- Vijayalakshmi et al. (2020) A Vijayalakshmi et al. Deep learning approach to detect malaria from microscopic images. Multimedia Tools and Applications, 79(21):15297–15317, 2020.
- Wang et al. (2020) Hongda Wang, Hatice Ceylan Koydemir, Yunzhe Qiu, Bijie Bai, Yibo Zhang, Yiyin Jin, Sabiha Tok, Enis Cagatay Yilmaz, Esin Gumustekin, Yair Rivenson, et al. Early-detection and classification of live bacteria using time-lapse coherent imaging and deep learning. arXiv preprint arXiv:2001.10695, 2020.
- Woolson (2007) RF Woolson. Wilcoxon signed-rank test. Wiley encyclopedia of clinical trials, pages 1–3, 2007.
- Xu et al. (2020) XF Xu, S Talbot, and T Selvaraja. Deep learning based cell parasites detection. arXiv preprint arXiv:2002.11327, 2020.
- Xue and Ray (2017) Yao Xue and Nilanjan Ray. Cell detection in microscopy images with deep convolutional neural network and compressed sensing. arXiv preprint arXiv:1708.03307, 2017.
- Yang et al. (2019) Feng Yang, Mahdieh Poostchi, Hang Yu, Zhou Zhou, Kamolrat Silamut, Jian Yu, Richard J Maude, Stefan Jaeger, and Sameer Antani. Deep learning for smartphone-based malaria parasite detection in thick blood smears. IEEE Journal of Biomedical and Health Informatics, 24(5):1427–1438, 2019.
- Zieliński et al. (2020) Bartosz Zieliński, Agnieszka Sroka-Oleksiak, Dawid Rymarczyk, Adam Piekarczyk, and Monika Brzychczy-Włoch. Deep learning approach to describe and classify fungi microscopic images. PloS one, 15(6):e0234806, 2020.
A Supplementary Information
A.1 Implementation Details
The networks were implemented in Python . The open source object detection library mmdetection (Chen et al., 2019) was used with ResNeXt101 backbone accompanied with feature pyramid network (FPN) for Faster RCNN (Nguyen et al., 2020), and ResNet101 backbone for RetinaNet. Similarly, YOLOv8s was implemented by cloning the repository of Ultralytics (Jocher et al., 2023). For Faster RCNN and RetinaNet, the shortest edge was resized with sharp edge lengths of 640, 472, 704, 736, 768, and 800, and a random horizontal flip with a probability of was used during data augmentation. For YOLOv8s, random horizontal flips were applied along with mosaic augmentation with probability and 1.0, respectively, for reference and sample images. We adapted the learning rate, iterations, and the number of classes for all three models with empirical experiments during training to get the optimal performance. Additionally, for RetinaNet, the focal loss’s alpha and gamma parameters were adjusted to improve the results from the default settings. The hyperparameters used for these three models are summarized Table 5.
Microscope | Detector | Backbone | W/N | LR | Other |
---|---|---|---|---|---|
Brightfield | Faster RCNN | ResNeXt101 | 1200/1500 | FPN, PPI = | |
RetinaNet | ResNet101 | 800/1200 | |||
YOLOv8s | CSPDarknet | 3/100 | batch size=16 | ||
Smartphone | Faster RCNN | ResNeXt101 | 1500/2000 | FPN, PPI = 64 | |
RetinaNet | ResNet101 | 1200/1500 | |||
YOLOv8s | CSPDarknet | 3/200 | batch size = 16 |
A.2 Statistical Analysis
The Quantile-Quantile (Q-Q) plot was used to check if the data were normally distributed. Since the data were not normally distributed, we selected paired Wilcoxon signed-rank test (Woolson, 2007) to test the significance between the predictions. A p-value of less than (i.e., ) was considered significant. We have assumed total images as the sample size (i.e., sample size = ) and the count of parasites on each image as the scores. Since the large sample size (), we used normal approximation on the Wilcoxon signed-rank test. Here, normal approximation does not mean the data distribution is normal, but the Wilcoxon signed rank test statistic is assumed to be approximately normal. We used scipy444https://docs.scipy.org/doc/scipy/reference/generated/scipy.stats.wilcoxon.html to perform the Wilcoxon signed-rank test.
The null hypothesis is that there is no difference between the cysts-count predictions between the non-experts and models. The p-values are provided in Table 6 for Giardia and Table 7 for Cryptosporidium. We observed significantly different results among all the non-experts and between Non-expert and models. All three models significantly outperformed the non-experts for the detection of Cryptosporidium. However, for Giardia, only Faster RCNN had a statistically significant difference in performance compared to Non-expert 1 and Non-expert 2. In contrast, RetinaNet had a statistically significant difference in performance compared to Non-expert 3. Faster RCNN and RetinaNet were significantly better than non-expert human in predicting Giardia and Cryptosporidium.
Expert | Non-expert 1 | Non-expert 2 | Non-expert 3 | Faster RCNN | RetinaNet | |
---|---|---|---|---|---|---|
Non-expert 1 | 1.64E-04 | |||||
Non-expert 2 | 4.72E-04 | 1.29E-08 | ||||
Non-expert 3 | 1.98E-04 | 4.04E-08 | 7.62E-01 | |||
Faster RCNN | 2.19E-09 | 2.60E-02 | 5.71E-13 | 7.88E-12 | ||
RetinaNet | 1.08E-09 | 2.59E-02 | 1.02E-13 | 2.60E-12 | 7.79E-01 | |
YOLOv8s | 1.10E-13 | 1.57E-05 | 5.18E-17 | 6.42E-15 | 4.50E-05 | 7.74E-05 |
Expert | Non-expert 1 | Non-expert 2 | Non-expert 3 | Faster RCNN | RetinaNet | |
---|---|---|---|---|---|---|
Non-expert 1 | 1.14E-04 | |||||
Non-expert 2 | 3.03E-21 | 5.52E-25 | ||||
Non-expert 3 | 9.37E-03 | 8.51E-10 | 8.88E-21 | |||
Faster RCNN | 5.60E-04 | 2.62E-02 | 1.86E-24 | 4.33E-09 | ||
RetinaNet | 1.37E-04 | 1.56E-01 | 3.52E-26 | 6.40E-10 | 3.23E-01 | |
YOLOv8s | 6.67E-02 | 8.97E-04 | 2.01E-23 | 1.82E-06 | 5.43E-03 | 6.33E-04 |
A.3 Choice of thresholds
To calculate the precision, recall, and f1-score of the object detection models, we plotted the precision-recall of the respective detectors for different values of iou thresholds and confidence scores, ranging from 0.1 to 1 with a step size of 0.1, as in Figure 4 Then, we selected the thresholds by observing the respective plot to get the best precision and recall.
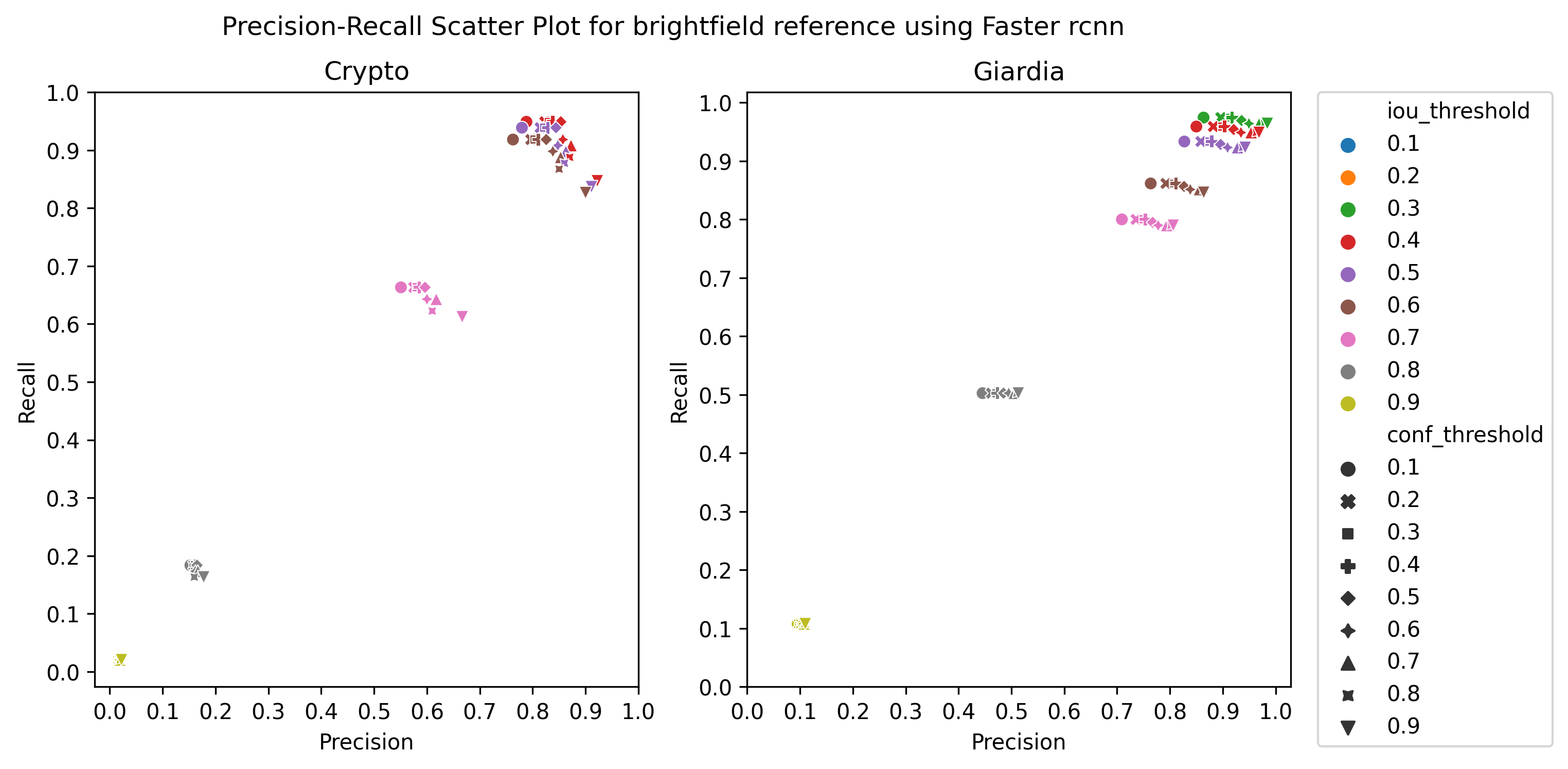
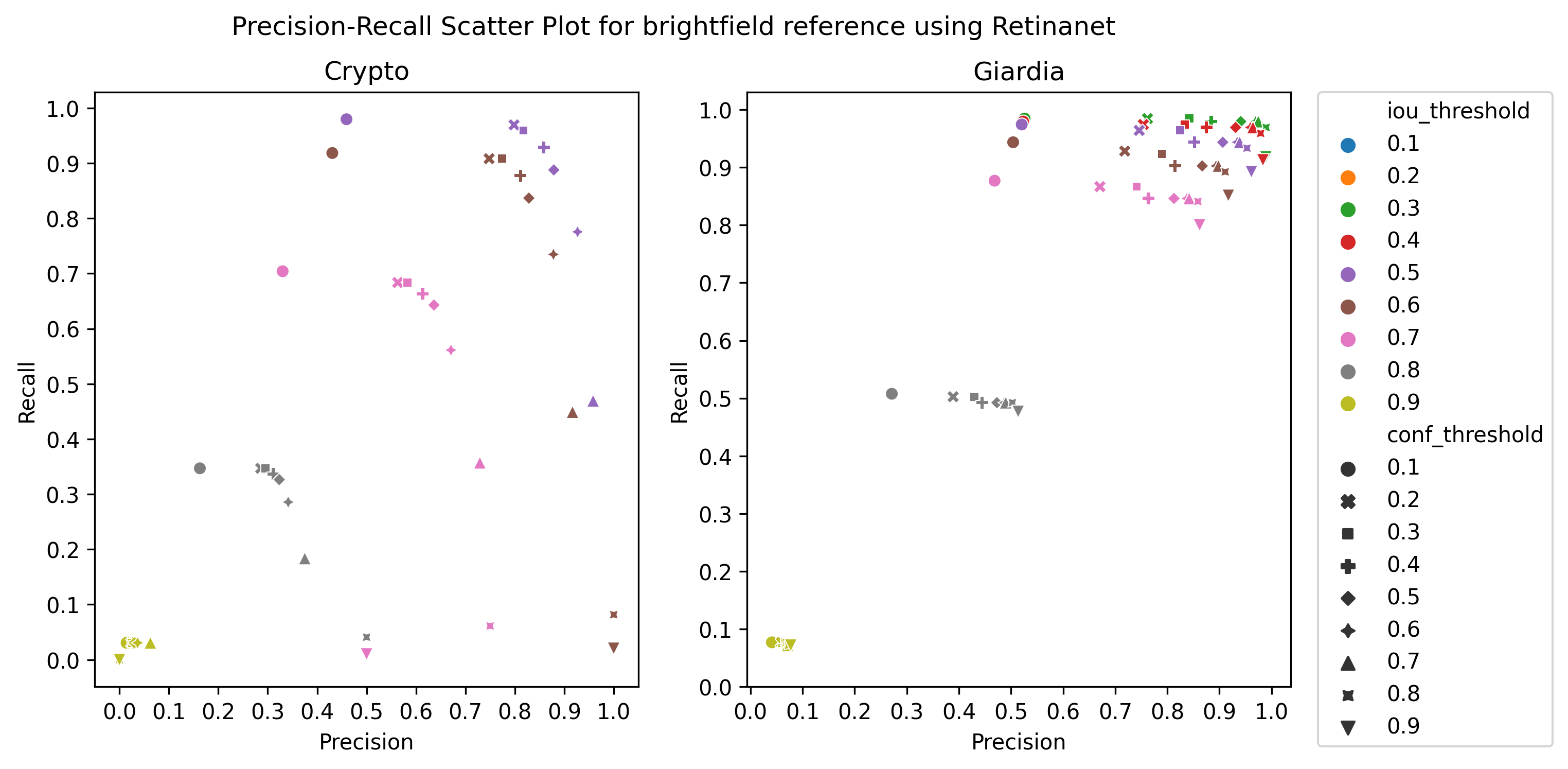
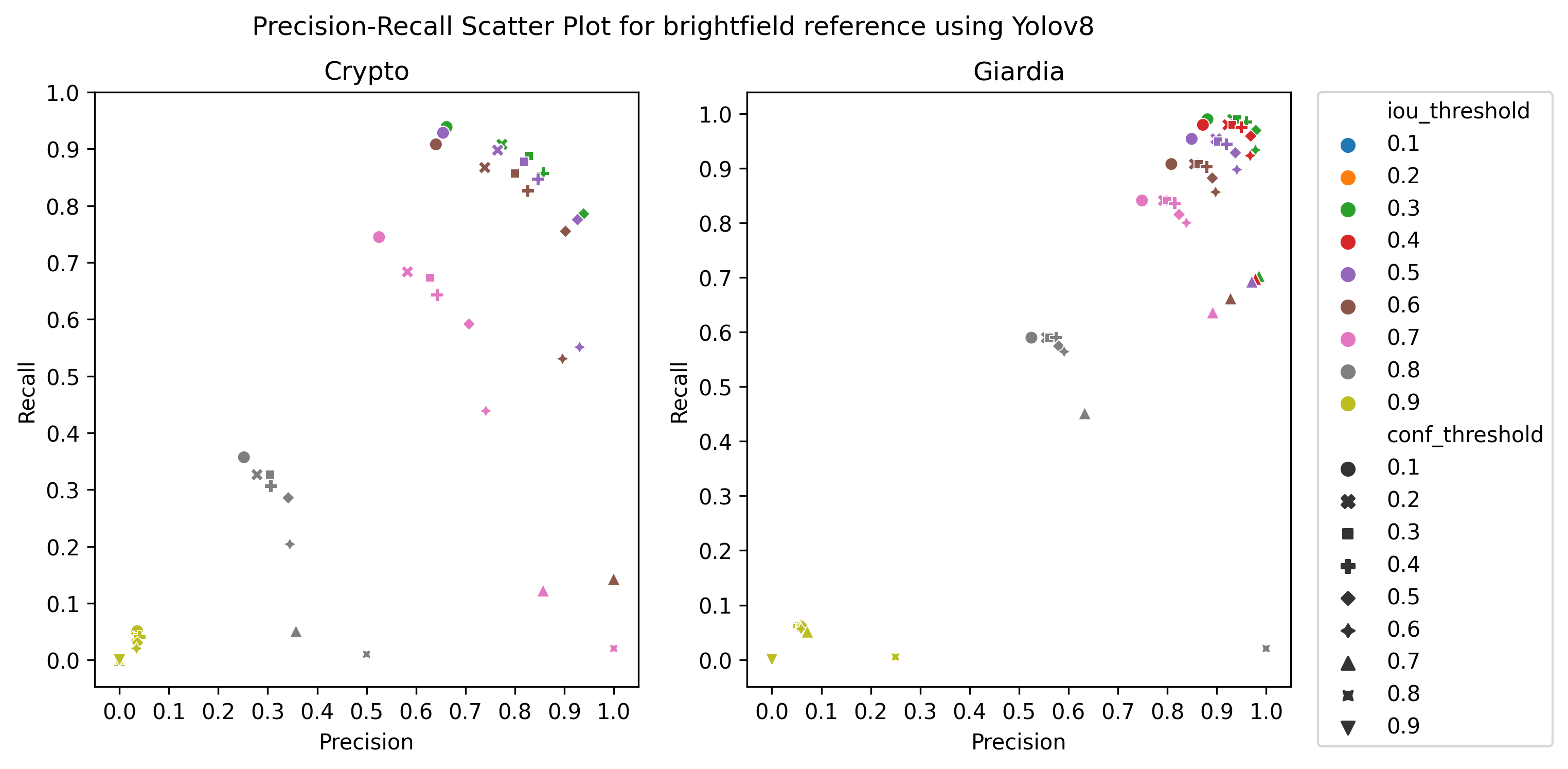
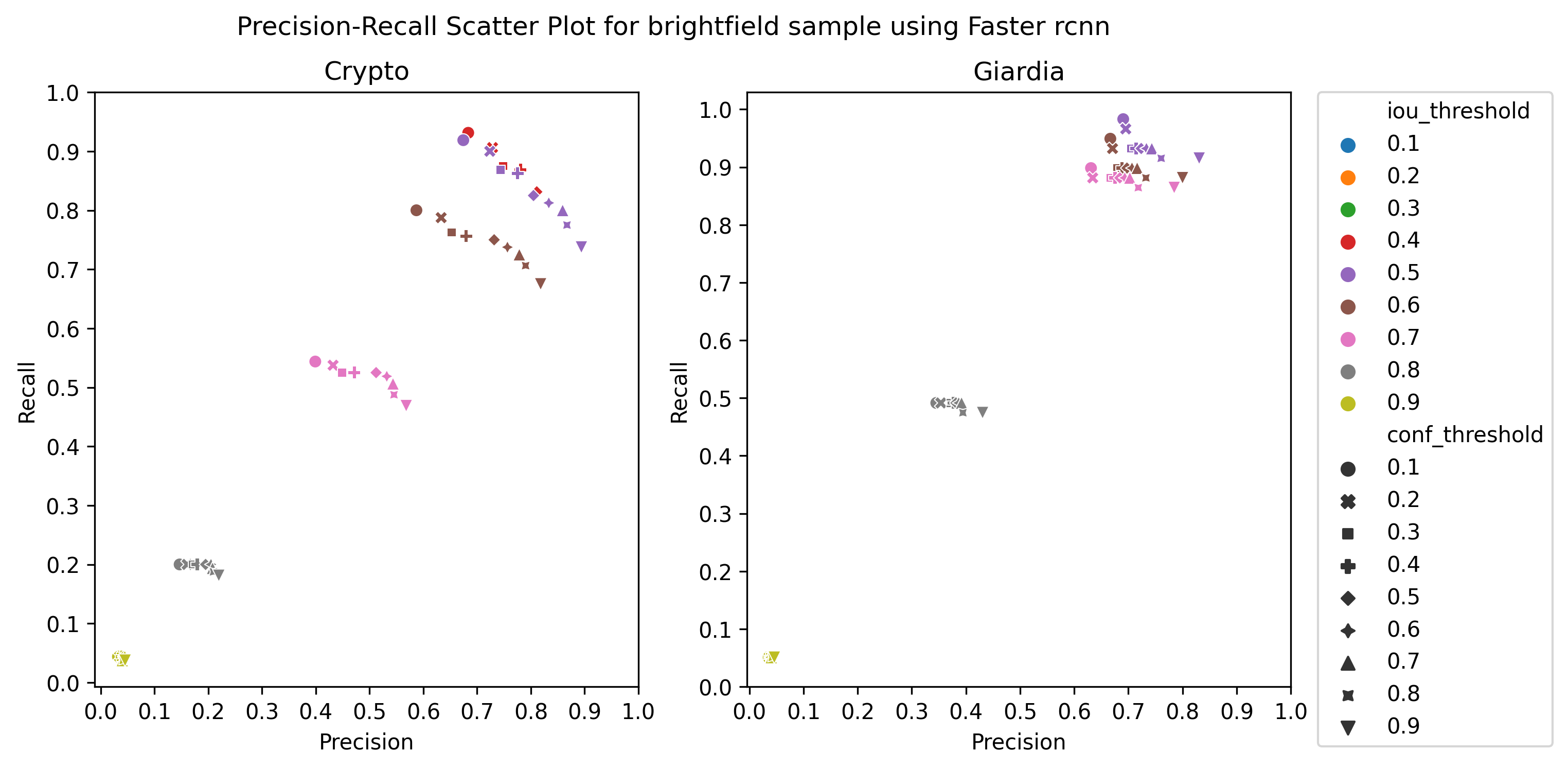
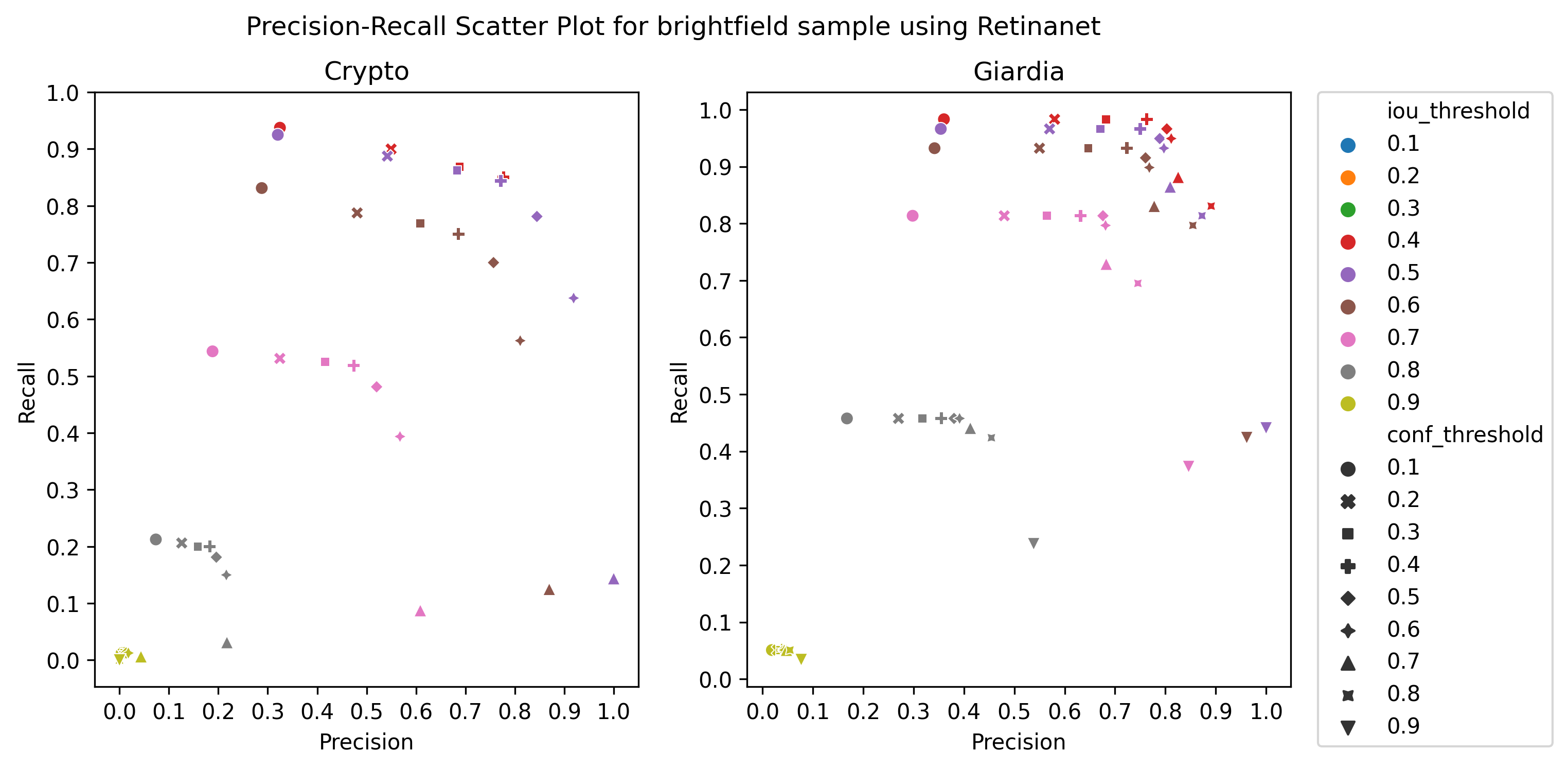
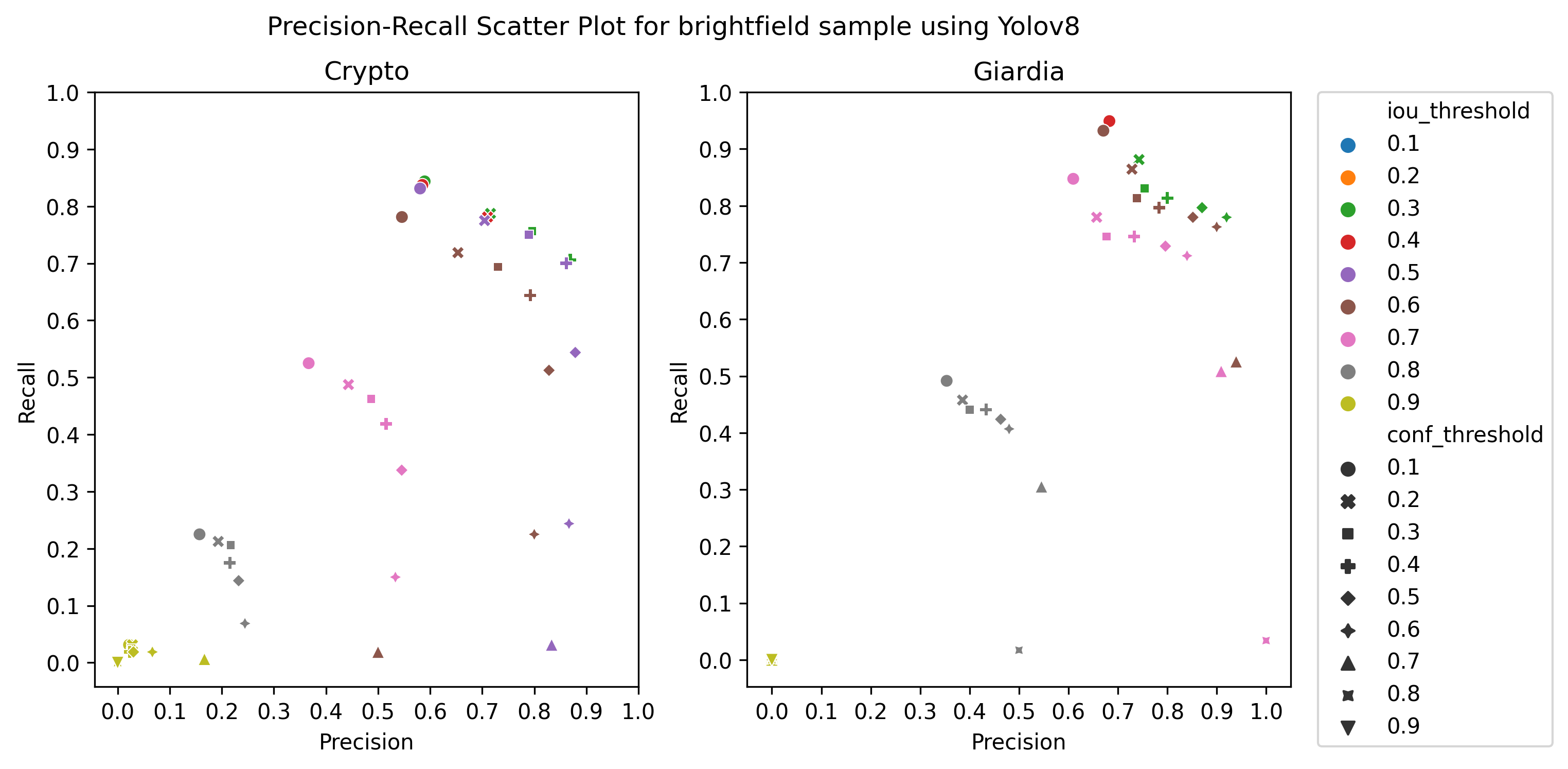
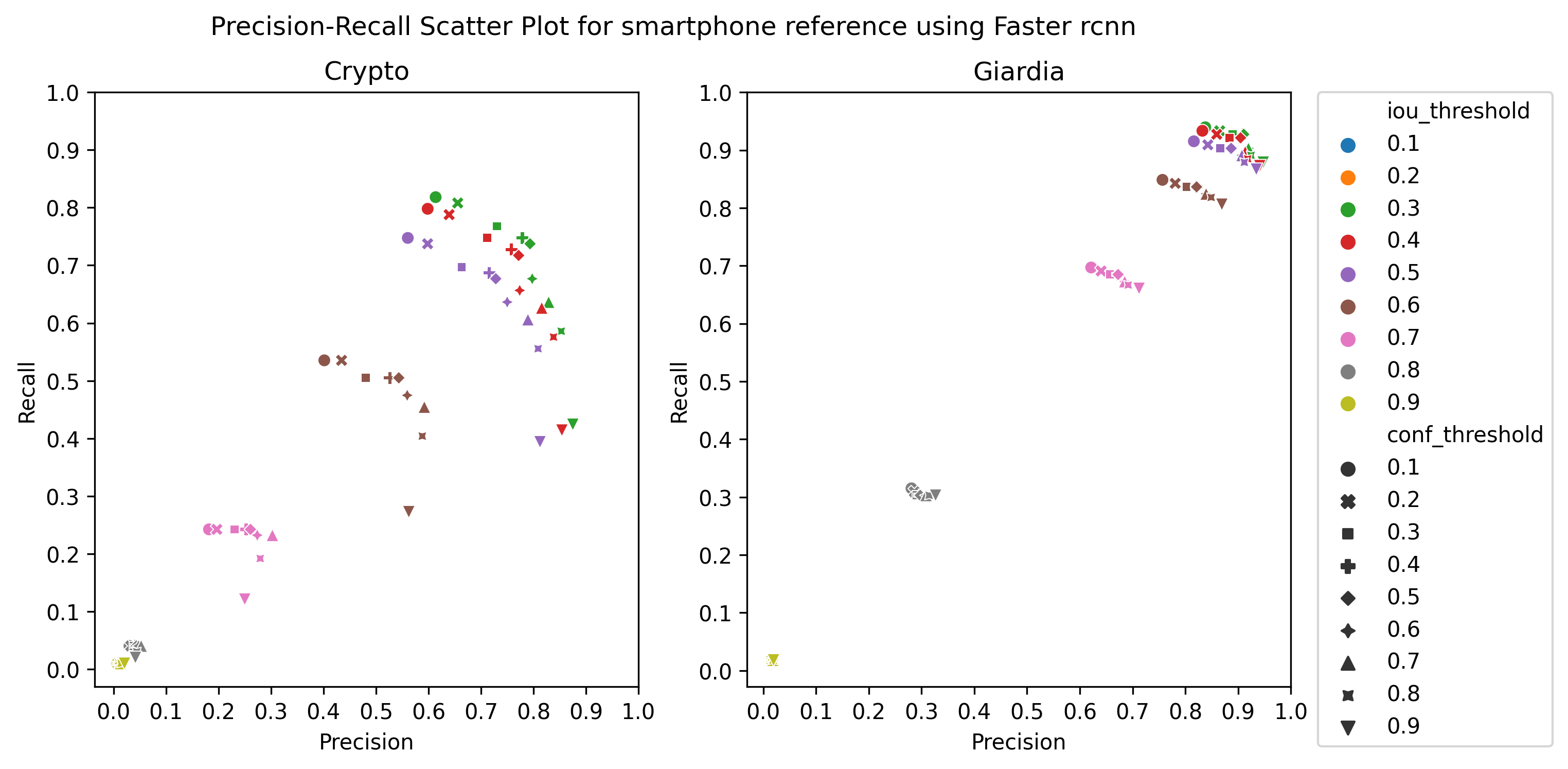
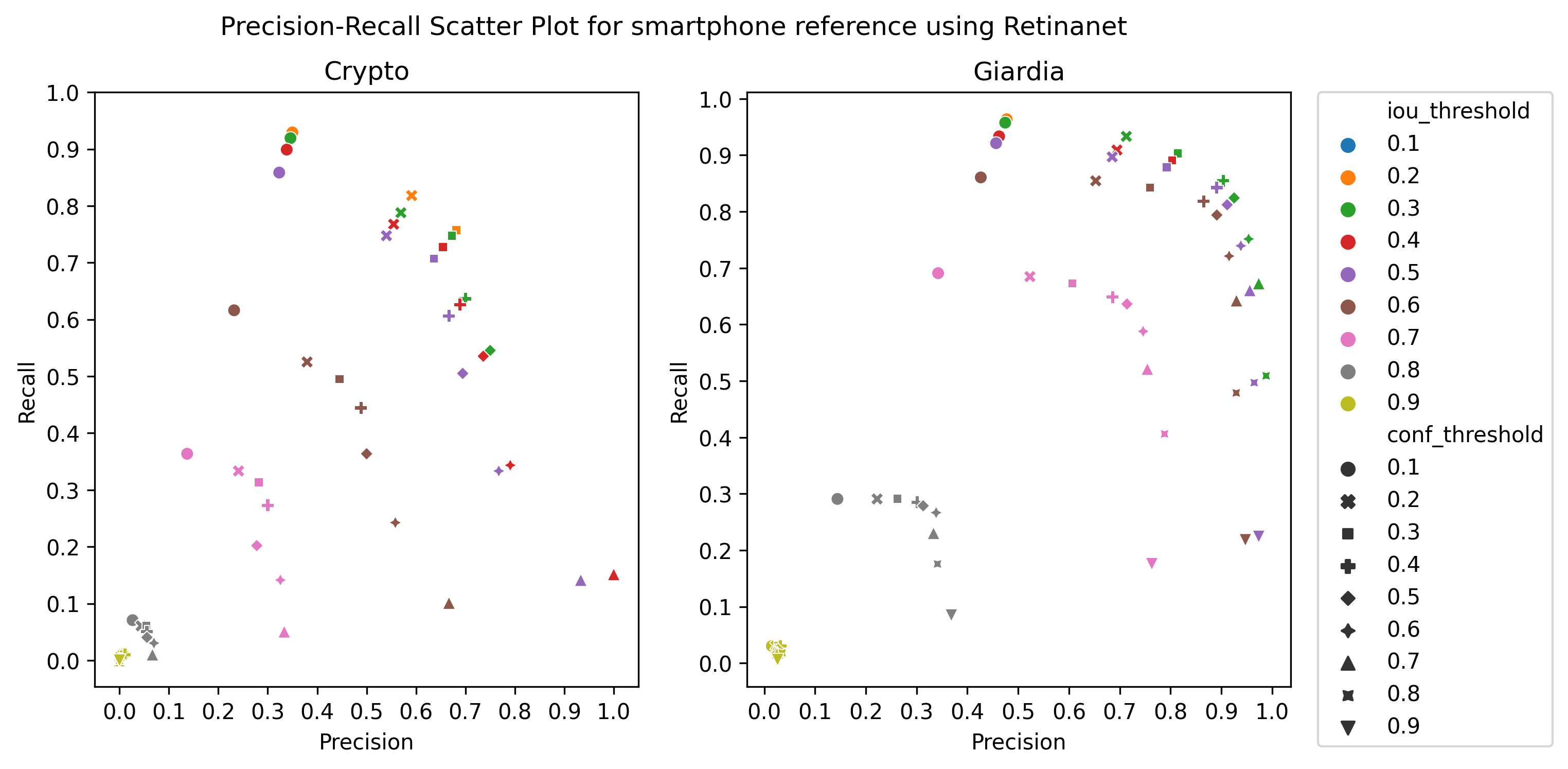
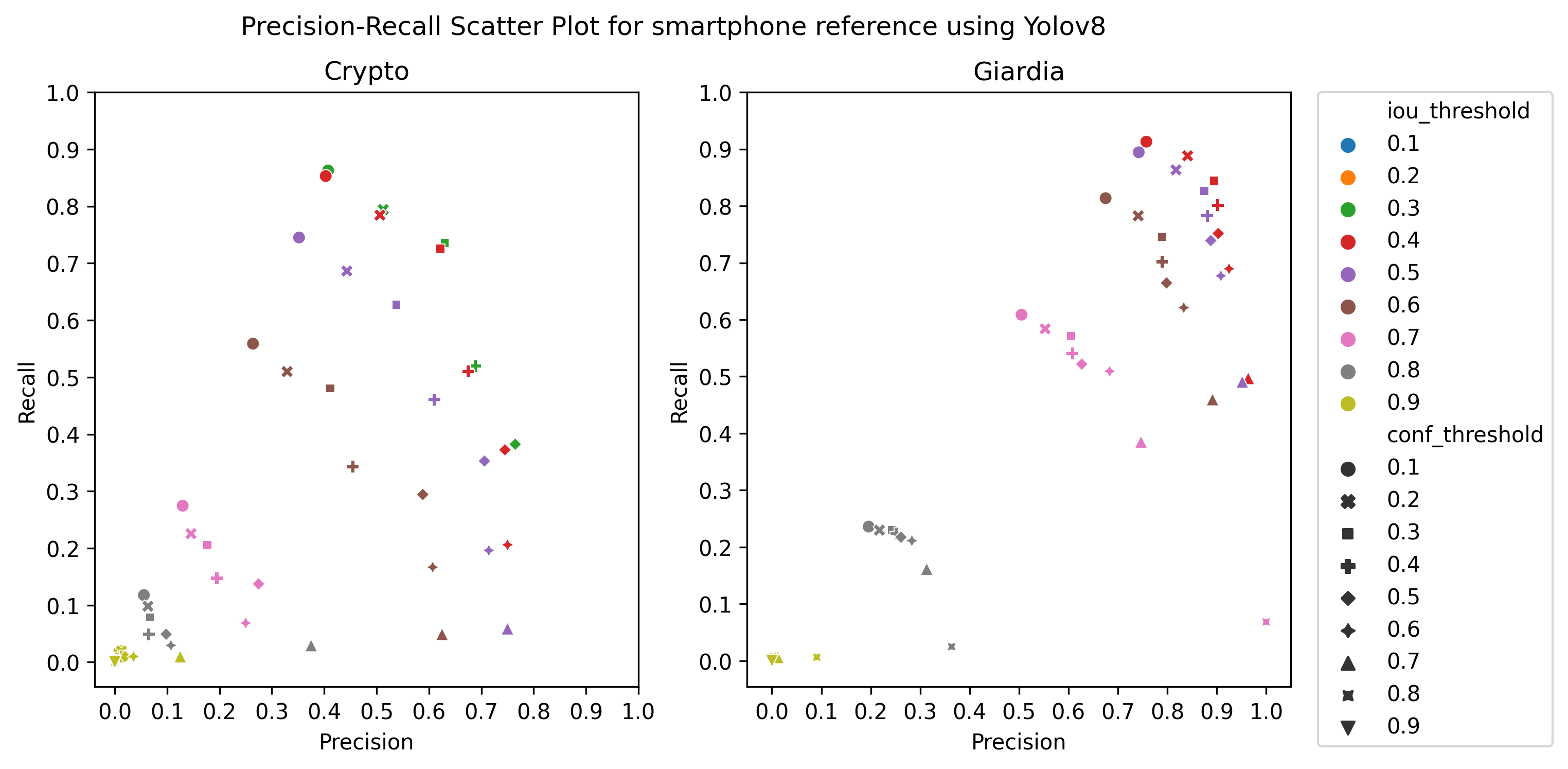
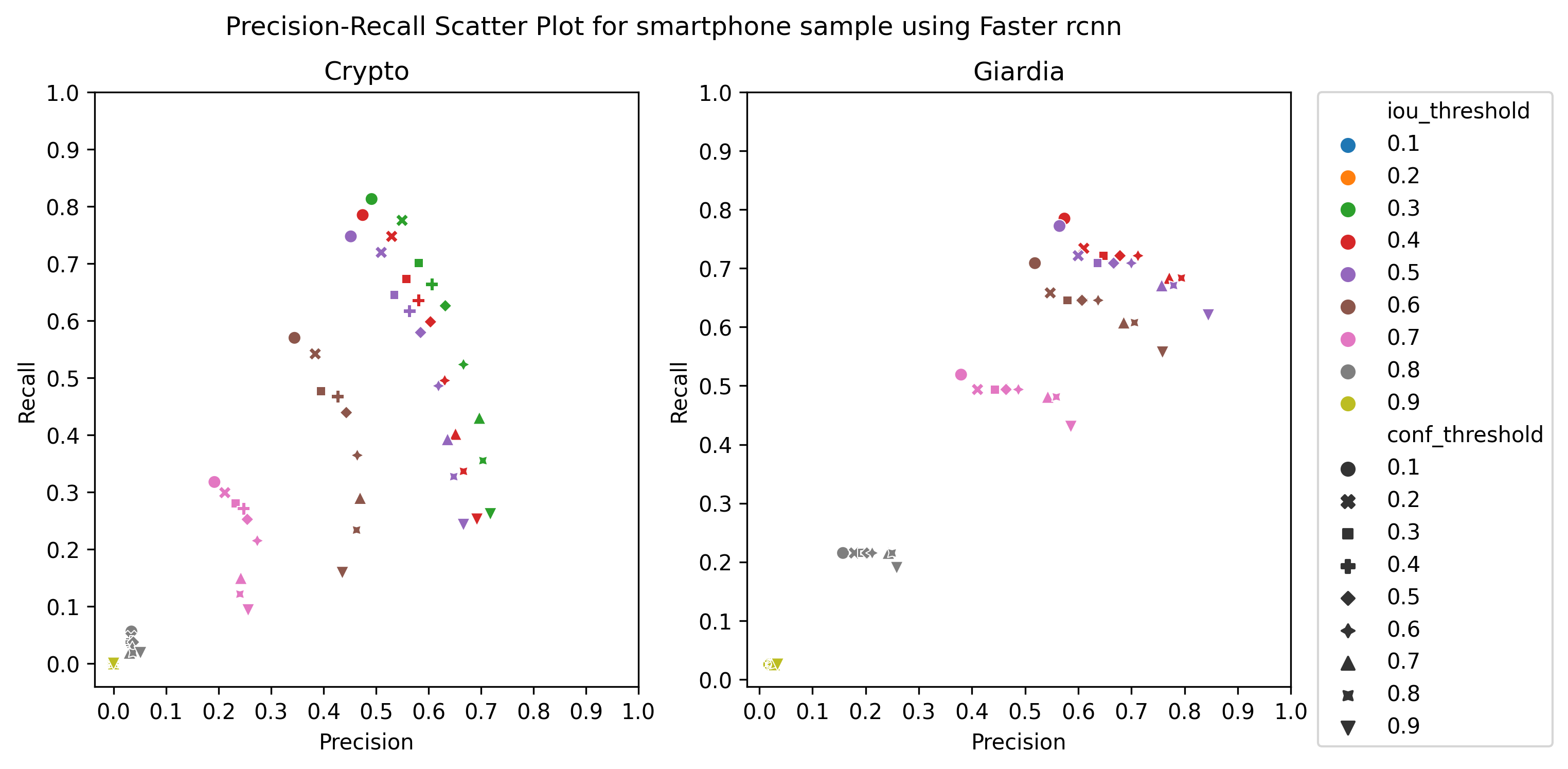
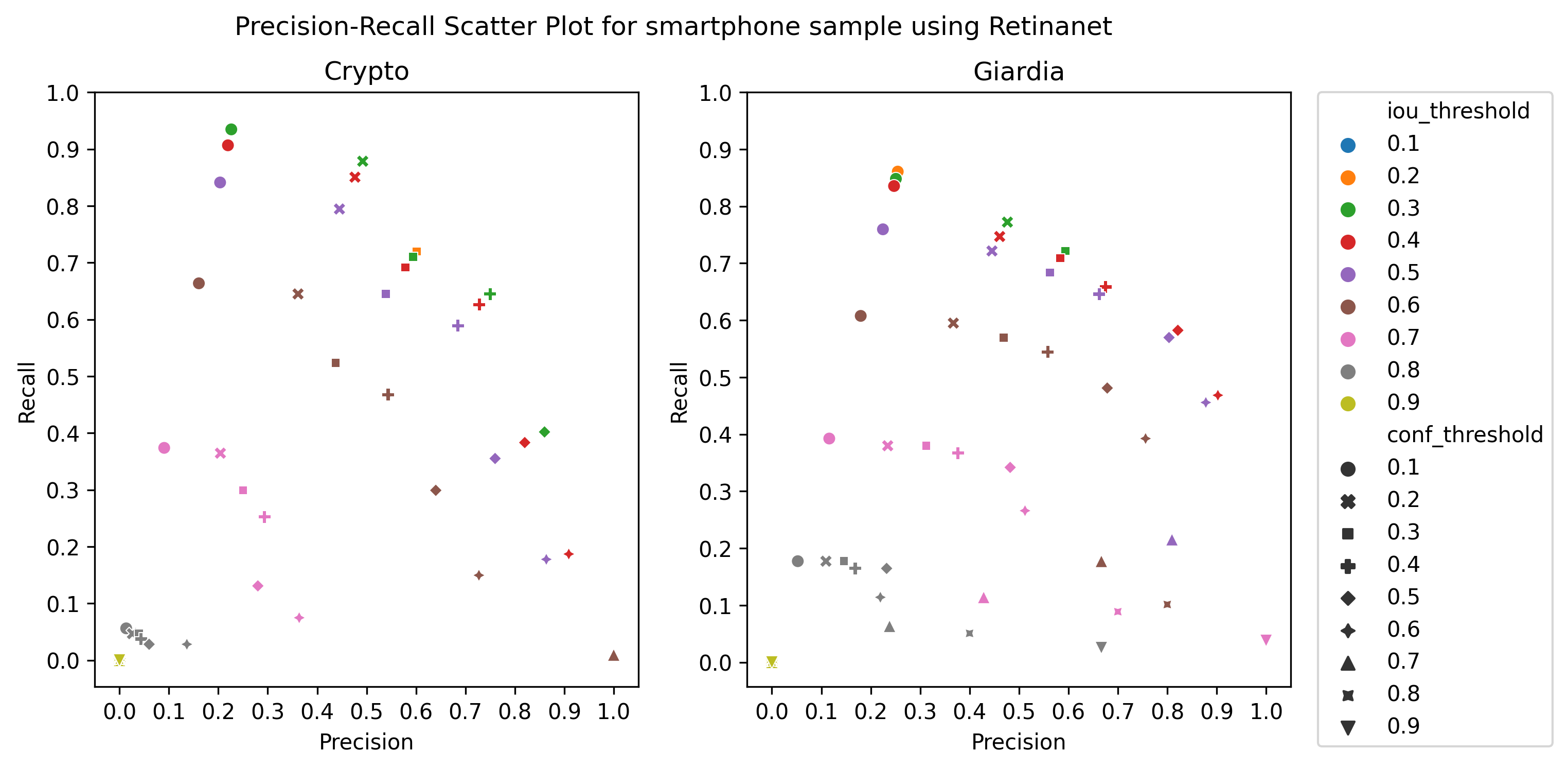
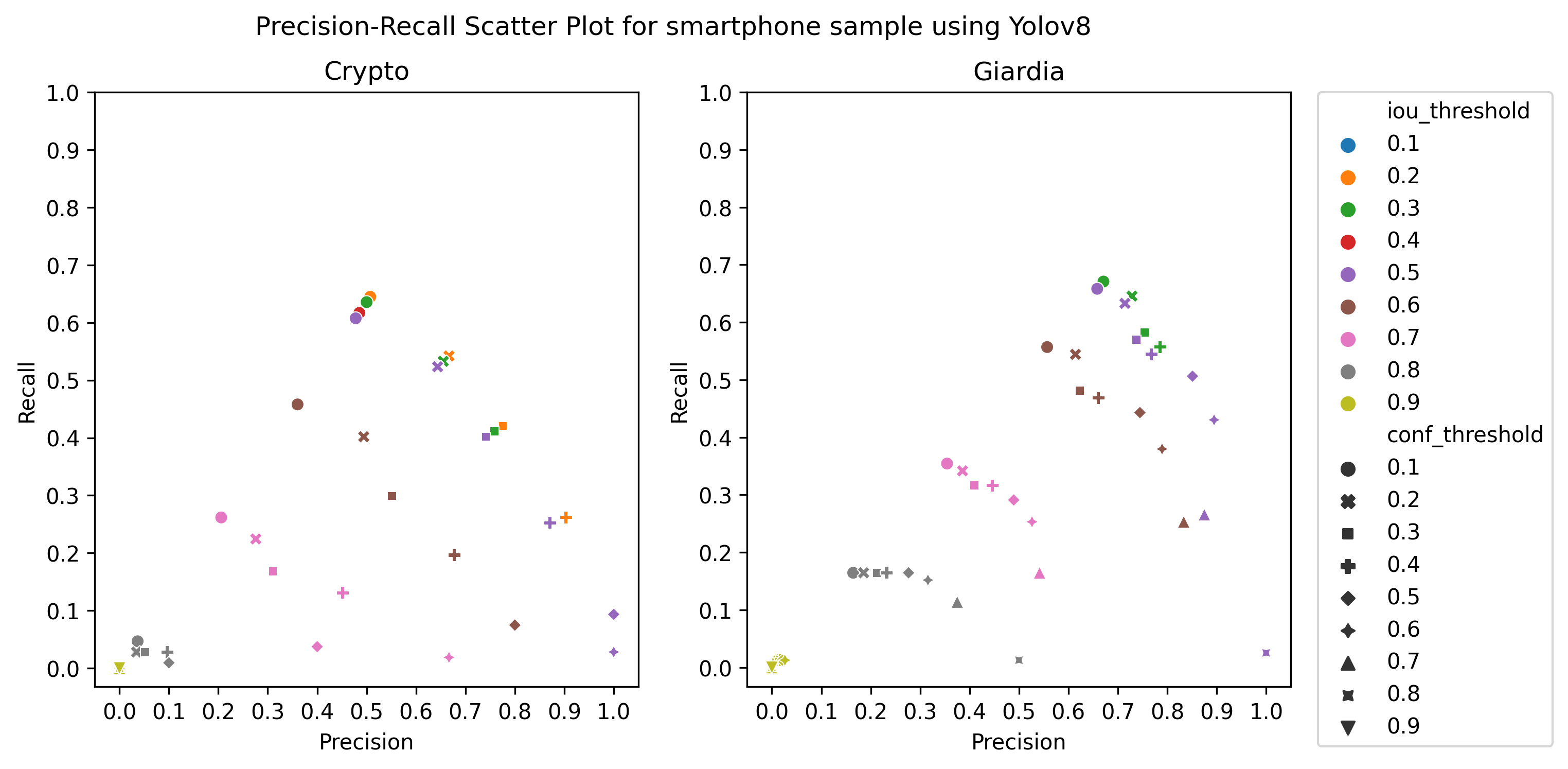